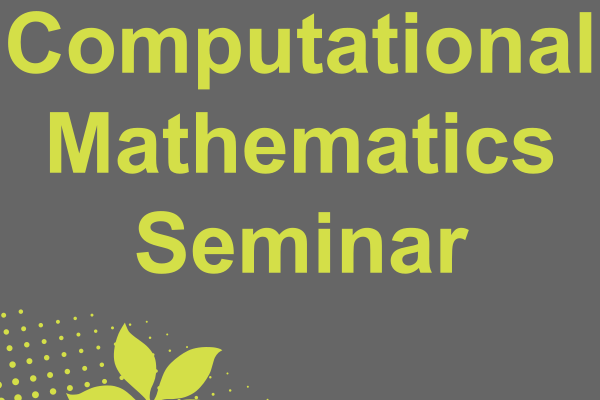
Speaker: Mrinal Kumar (OSU)
Title: Adaptive Model Learning and Uncertainty Forecasting in Complex Dynamic Systems
Abstract: This talk will begin with an overview of the research at the Laboratory for Data-Driven and Complex Systems (LADDCS) in the MAE Department at The Ohio State University. We will briefly discuss our work on the integration of uncrewed aerial systems (UAS) in wildland fire management with focus on some interesting resource-constrained path planning problems. The talk will then move towards the coupled problem of learning dynamic systems, and the propagation of uncertainty through them. A long-standing problem in nonlinear dynamic systems is the propagation of uncertainty through such systems, governed by the Fokker-Planck equation (FPE). This problem suffers from the triple curses of dimensionality, nonlinearity, and resultant non-Gaussianity. An important application of FPE is in space domain awareness (SDA), where trustworthy simulations of orbital mechanics are necessary for reliable decision-making concerning defensive space control. We will walk through a wide range of methods for solving the FPE developed over the past half century, and our own efforts over the past decade+, spanning discretization techniques such as partition of unity FEM, tensor methods, and particle methods. We will focus attention on a novel adaptive Monte Carlo (AMC) approach that draws insights from Markov chain Monte Carlo (MCMC) to answer the following central question – how many particles are necessary to meet a prescribed forecasting accuracy in a user-defined quantity of interest (QoI). A closed-loop architecture for Monte Carlo will be presented, which tracks its forecasting performance vis-à-vis prescribed QoI accuracy. When excess error is detected, it adds optimal new particles to the simulation, identified by solving global optimization of the discrepancy function at the initial time. Challenges and open questions related to optimization of the discrepancy function will be discussed. Finally, we will point out that performance guarantees of the closed loop AMC platform are contingent on the knowledge of the dynamic model of the underlying physical process. When this condition is not met, the AMC platform offers the opportunity to iteratively learn process dynamics in an outer loop either in a physics-based or a data-driven form iteratively using residual forecasting errors. Several interesting applications, including SDA will be discussed along the way.