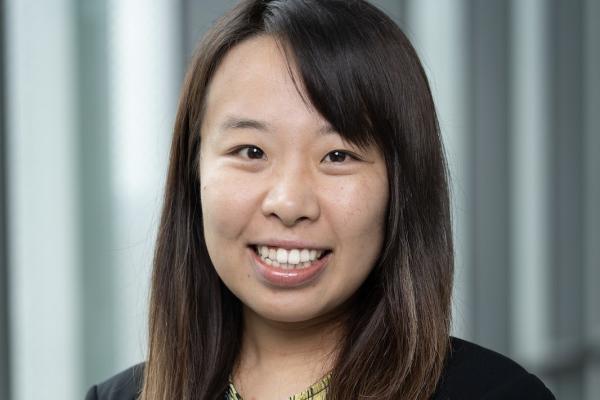
Yuanyuan Shi
UCSD
Title
Neural Operator Learning for PDE Control
Abstract
Performing real-time state estimation and control for PDEs using provably and rapidly converging observers and controllers, such as those based on PDE backstepping, is computationally expensive and in many cases prohibitive. We propose a framework for accelerating PDE control using learning-based approaches that are much faster while maintaining closed-loop stability. In particular, we employ Neural Operators to learn the functional mapping from the boundary measurements to the state estimate and boundary control. By employing backstepping gains for model-based controllers and observers with particular convergence rate guarantees, we provide numerical experiments that evaluate the increased computational efficiency gained with neural operators. The ML-accelerated methods can achieve up to three orders of magnitude improvement in computational speed compared to classical methods. This demonstrates the attractiveness of the ML-accelerated methods combined with knowledge about the physical systems for real-time PDE system state estimation and control.