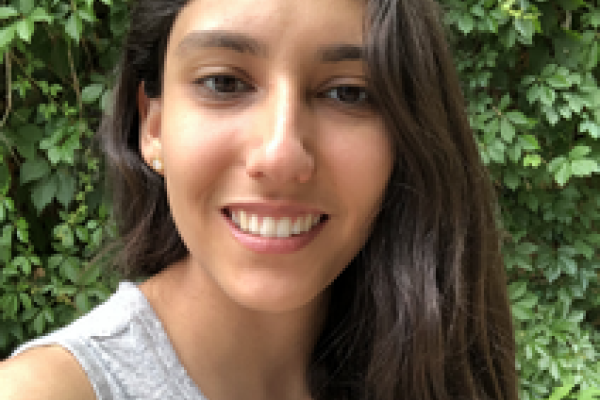
Title: Nonnegative Low-Dimensional Representations in Learning Tasks
Speaker: Lara Kassab (UCLA)
Speaker's URL: https://larakassab.weebly.com
Abstract: With the ever-increasing access to data, one of the greatest challenges that remains is how to make sense out of this abundance of information. In this introductory talk, we present mathematical methods based on matrix and tensor factorization for machine learning tasks.
First, supervision-aware dimensionality-reduction models have become increasingly important in data analysis; such models aim to use supervision in the process of learning the lower-dimensional representation. A popular technique for topic modeling that provides a lower rank approximation of a matrix is nonnegative matrix factorization (NMF). We propose variants of semi-supervised NMF models that provide a low-dimensional representation (topic model) and a model for classification.
Second, although many datasets can be represented as matrices, datasets also often arise as high-dimensional arrays, known as higher-order tensors. We show that nonnegative CANDECOMP/PARAFAC tensor decomposition successfully detects short-lasting topics in temporal text datasets that other methods fail to fully detect.
URL associated with Seminar: https://tgda.osu.edu/activities/tdga-seminar/