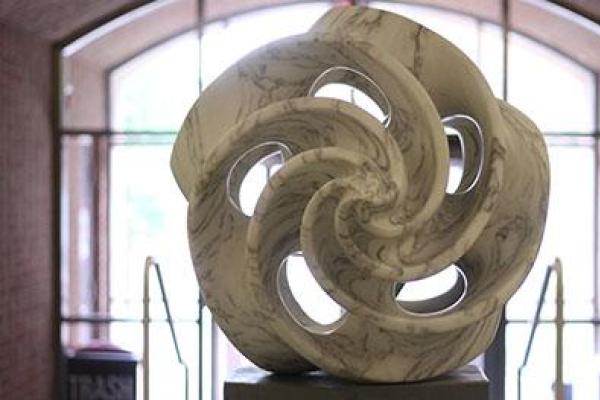
Title: Privacy-Preserving Collaborative Information Sharing through Federated Learning - A Case of the Insurance Industry
Speaker: Zhiyu Quan (University of Illinois at Urbana-Champaign)
Abstract: This research explores the critical balance between leveraging proprietary data for collaborative gains and protecting business-sensitive information and client privacy. Taking advantage of unique and comprehensive proprietary data from multiple insurance and InsurTech firms, we present the first comprehensive examination of the real economic benefits of the federated learning framework as a privacy-enhancing business solution for data collaboration. We cover a complete variety of dimensions across the possibilities of boundary-spanning multiple business entities in the business context. The proposed business solution could foster access to the collective intelligence of industry peers in scenarios where physical data aggregation is infeasible, thereby yielding a more comprehensive assessment of user-related features across entities and providing industry-level insights, enhancing standards, preventing fraud, improving efficiency, and driving innovation.