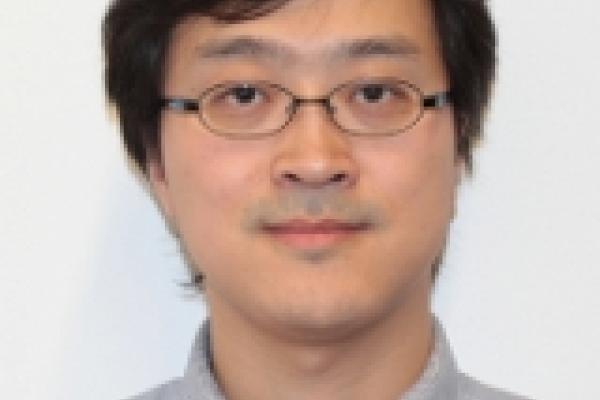
Title: Computing Critical Points of a High Dimensional Distribution via Graphical Models
Speaker: Chao Chen (CUNY)
Abstract: The computation of persistent homology is challenging for high dimensional data. In this talk, I will discuss an alternative direction that I am currently pursuing. The idea starts with representing the high dimensional data using a concise probabilistic model, namely, the graphical model. Next, we directly compute the critical points on the underlying graph instead of building a discretization of the domain. I will discuss different methods, e.g., dynamic programming, heuristic search and local neighborhood search, to compute local maxima and their attractive basins. I will also discuss the computation of saddle points. These methods have been used in making multiple diverse predictions and in clustering.
Seminar URL: https://research.math.osu.edu/tgda/tgda-seminar.html