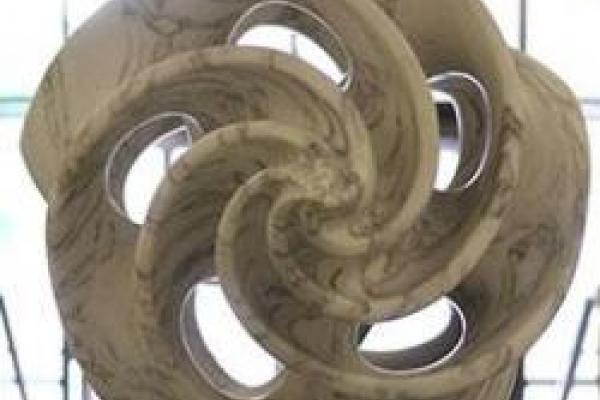
Title: Deep Online Convex Optimization with Gated Games
Speaker: David Balduzzi (Victoria University, New Zealand)
Abstract:The most powerful class of feedforward neural networks are rectifier networks which are neither smooth nor convex. Standard convergence guarantees from the literature therefore do not apply to rectifier networks.
In this talk, I will describe a game-theoretic analysis of rectifier neural networks that leads to a simple convergence proof based on the notion of correlated equilibrium. The key technical tool is to express error backpropagation using path-sums, a formalism that is interesting in its own right. A large fraction of the talk will be an introduction to neural nets, starting from scratch.