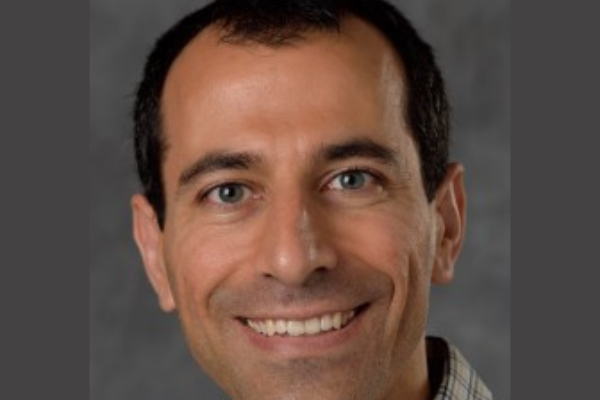
Title: Topological Data Analysis of Time Series
Speaker: Firas Khasawneh (Michigan State University)
Abstract: This talk describes recent applications of some tools from Topological Data Analysis (TDA) for studying dynamical systems. Specifically, we describe two examples where TDA provides useful tools for understanding the underlying system dynamics:
- Chatter identification in machining by combining supervised machine learning with TDA. This work is motivated by the difficulty in defining a metric that captures the characteristics of chatter or a threshold that signals its occurrence. In traditional chatter detection methods, this difficulty can be somewhat alleviated by utilizing machine learning techniques. However, even with a successful classification algorithm, the transferability of typical machine learning methods from one data set to another remains very limited. In this talk we show how combining supervised machine learning with TDA can lead to a powerful classification algorithm. The features we use are derived from the persistence diagram of an attractor reconstructed from the time series via Takens embedding. We test the approach using simulated deterministic and stochastic turning models. Our results show a 97% successful classification rate on the deterministic model. Further, the results demonstrate that the resulting classifier can provide guidance on the stability regions in the stochastic setting where it is much more difficult to detect chatter.
- Detection of the true steps in piecewise constant (PWC) signals. The signal in question is a two-state square wave with randomly varying in-between-pulse spacing, and subject to spurious steps at the rising or falling edges which we refer to as digital ringing. We use persistent homology to derive mathematical guarantees for the resulting change detection which enables accurate identification and counting of the true pulses. The approach is described and tested using both synthetic and experimental data obtained using an engine lathe instrumented with a laser tachometer. We will show that the described approach significantly outperforms Fourier analysis when the spacing between the peaks is varied. We also generalize the approach to higher dimensional PWC signals, although utilizing this extension remains an interesting question for future research.
Biosketch: Dr. Firas Khasawneh is an Assistant Professor of Mechanical Engineering at Michigan State University. Prior to joining MSU, Dr. Khasawneh was an assistant professor of Mechanical Engineering at SUNY Polytechnic Institute in Utica, NY from 2013 to 2017. He received his PhD in 2010 from Duke University, and his Masters in 2007 from the University of Missouri-Columbia. His areas of expertise include nonlinear dynamics and vibrations, machining processes, and new approaches to time series analysis. Dr. Khasawneh is a long-time member of both ASME and SME, and his research is funded by the National Science Foundation.
Seminar URL: https://research.math.osu.edu/tgda/