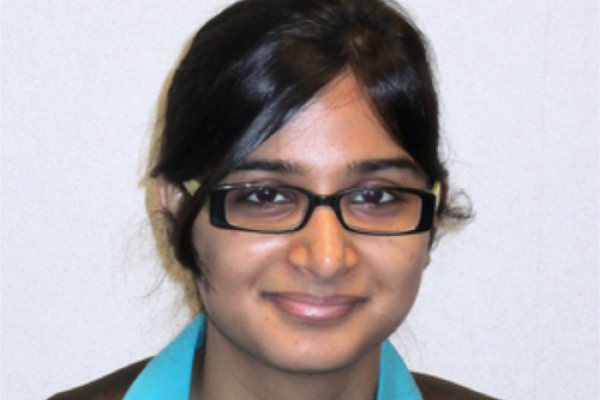
Title: Sketching and Clustering Metric Measure Spaces
Speaker: Kritika Singhal (Ohio State University)
Abstract: Two of the most fundamental data analysis tasks are clustering and simplification (sketching) of data. Clustering refers to partitioning a dataset, according to some rule, into sets of smaller size with the aim of extracting important information from the data. Sketching, or simplification of data, refers to approximating the input data with another dataset of much smaller size, in such a way that properties of the input dataset are retained by the smaller dataset. In this sense, sketching facilitates understanding of the data. There are many clustering methods for metric spaces and metric measure spaces (mm spaces) already present in literature, such as $k$-center clustering, $k$-median clustering, $k$-means clustering, etc. A natural method for obtaining a $k$-sketch of a metric space (mm space) is by viewing the space of all metric spaces (mm space) as a metric under Gromov-Hausdorff (Gromov-Wasserstein) distance, and then determining, under this distance, the $k$ point metric space (mm space) closest to the input metric space (mm space).
These two problems of sketching and clustering, a priori, look completely unrelated. However, we establish a duality i.e. an equivalence between these notions of sketching and clustering. I will describe the equivalence results obtained for the case of both metric spaces and metric measure spaces. For metric spaces, we consider the case where the clustering objective is minimizing the maximum cluster diameter. We show that the ratio between the sketching and clustering objectives is constant over compact metric spaces. For metric measure spaces, we prove that the ratio of sketching to clustering objectives is bounded both above and below by some universal constants. In this setting, the clustering objective involves minimizing various notions of the \emph{$\ell_p$-diameters} of the clusters. We consider two competing notions of sketching for metric measure spaces, with one of them being more demanding than the other. These notions arise from two different definitions of $p$-Gromov-Wasserstein distance that have appeared in the literature.
We also identify procedures/maps that transform a solution of the sketching problem to a solution of the clustering problem, and vice-versa. These maps give rise to algorithms for performing these transformations and, by virtue of these algorithms, we are able to obtain an approximation to the $k$-sketch of a metric measure space (metric space) using known approximation algorithms for the respective clustering objectives. I will briefly talk about these algorithms. This work is available online at https://arxiv.org/abs/1801.00551.
Seminar URL: https://tgda.osu.edu/