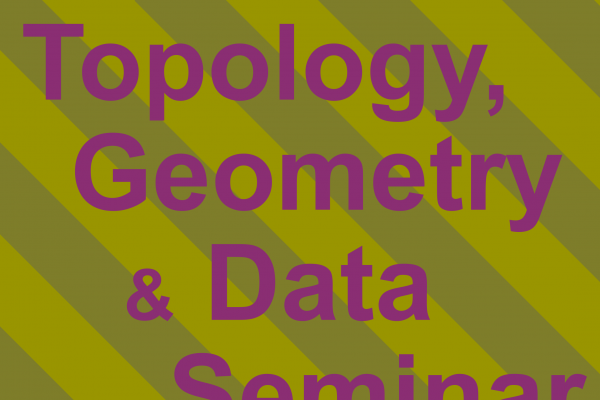
Title: Capturing Dynamics of Time-Varying Data via Topology
Speaker: Lori Ziegelmeier - Macalester College
Abstract: One approach to understanding complex data is to study its shape through the lens of algebraic topology. While the early development of topological data analysis focused primarily on static data, in recent years, theoretical and applied studies have turned to data that varies in time. A time-varying collection of metric spaces as formed, for example, by a moving school of fish or flock of birds, can contain a vast amount of information. There is often a need to simplify or summarize the dynamic behavior. One such method is a crocker plot, a 2-dimensional image that displays the (non-persistent but varying with scale) topological information at all times simultaneously. We use this method to perform exploratory data analysis and investigate parameter recovery via machine learning in the collective motion model of D’Orsogna et al. (2006). Then, we use it to choose between unbiased correlated random walk models of Nilsen et al. (2013) that describe motion tracking experiments on pea aphids. We then introduce a new tool to summarize time-varying metric spaces: a crocker stack. Crocker stacks are convenient for visualization, amenable to machine learning, and satisfy a stability property.