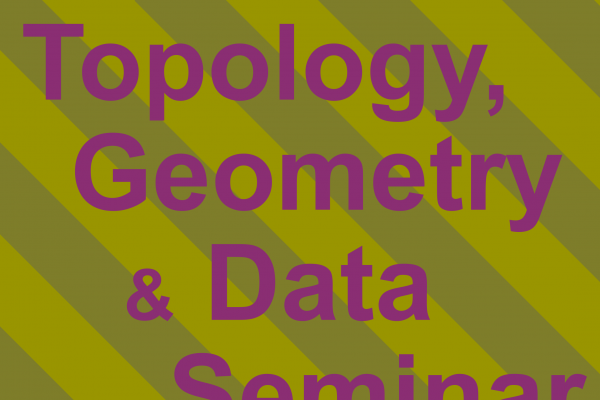
Title: Local cliques in ER-perturbed random geometric graphs
Speaker: Minghao Tian (Ohio State University, CSE)
Abstract: Random graphs are mathematical models that have applications in a wide range of domains. Among them, the Erdös--Rényi and the random geometric graphs are two models whose theoretical properties (e.g., clique number and the largest component) are perhaps most well studied. We are interested in mixed models coming from the overlay of two graph structures. In particular, we study the following model where one adds Erdös--Rényi (ER) type perturbation to a random geometric graph. More precisely, assume $G_\mathcal{X}^{*}$ is a random geometric graph sampled from a nice measure on a metric space $\mathcal{X} = (X,d)$. The input observed graph $\widehat{G}(p,q)$ is generated by removing each existing edge from $G_\mathcal{X}^*$ with probability $p$, while inserting each non-existent edge to $G_\mathcal{X}^{*}$ with probability $q$. We refer to such random $p$-deletion and $q$-insertion as ER-perturbation. Although these graphs are related to the objects in the continuum percolation theory, our understanding of them is still rather limited.
We consider a localized version of the classical notion of clique number for the aforementioned ER-perturbed random geometric graphs: Specifically, we study the \emph{edge clique number} for each edge in a graph, defined as the size of the largest clique(s) in the graph containing that edge. The clique number of the graph is simply the largest edge clique number. Interestingly, given a ER-perturbed random geometric graph, we show that the edge clique number presents two fundamentally different types of behaviors, depending on which ``type'' of randomness it is generated from.
As an application of the above results, we show that by using a filtering process based on the edge clique number, we can recover the shortest-path metric of the random geometric graph $G_\mathcal{X}^*$ within a multiplicative factor of $3$, from an ER-perturbed observed graph $\widehat{G}(p,q)$, for a significantly wider range of insertion probability $q$ than in previous work.
This is joint work with Matthew Kahle and Yusu Wang.