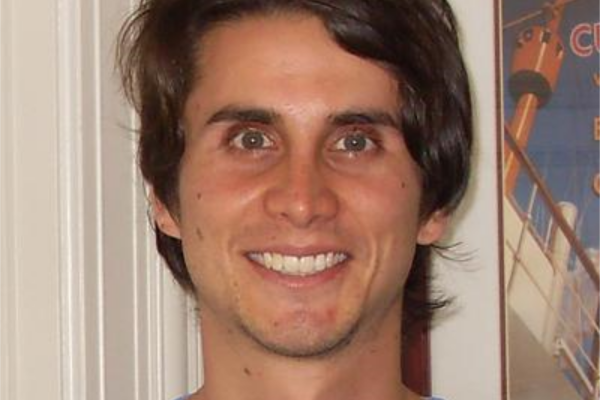
Title: Graph-based Bayesian learning: continuum limits and scalability of sampling algorithms.
Speaker: Nicolas Garcia-Trillos (Brown University)
Abstract: The principled learning of functions from data is at the core of statistics, machine learning and artificial intelligence. In this talk I will focus on Bayesian approaches to learning in the context of inverse problems on unknown domains and in particular semi-supervised learning. More specifically, we consider the problem of recovering a function input of a partial differential equation formulated on an unknown manifold $M$ where we can define a ground-truth Bayesian inverse problem. We assume to have access to a discrete domain $M_n = \{x_1, ... , x_n\} \subset M$, and to noisy measurements of the output solution at $p \leq n$ of those points. The discrete domain is then endowed with a graph structure which is used to create a graph surrogate for the ground-truth Bayesian inverse problem. The first theoretical result that I will present establishes the convergence of the graph posterior distribution associated to the graph-Bayesian inverse problem towards its ground-truth counterpart as the number of unlabeled data points converges to infinity. Our analysis relies on the variational description of posterior distributions, the choice of an appropriate topology to compare distributions on different domains, and precise quantitative estimates of the spectrum of graph Laplacians. I will then show that our consistency results have profound algorithmic implications: when they hold, carefully designed graph-based Markov chain Monte Carlo (MCMC) algorithms have a uniform spectral gap, independent of the number of unlabeled data. Several numerical experiments corroborate both the statistical consistency and the algorithmic scalability, and pave the way for future theoretical developments in the area of robust uncertainty quantification for machine learning tasks. This talk is based on works with Zachary Kaplan, Thabo Samakhoana, and Daniel Sanz-Alonso.
Seminar URL: https://research.math.osu.edu/tgda/tgda-seminar.html