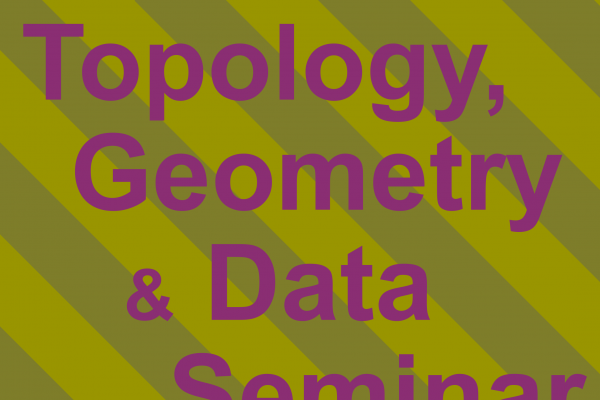
Title: Utilizing Topological Hierarchical Decomposition as a Functional Artificial Intelligence Unit for the Construction of Deep Topo
Speaker: Ryan Kramer (Air Force Research Laboratory)
Abstract: The rapid maturation of deep learning approaches has created a paradigm shift that intends to exponentially increase data exploitation capabilities across multiple domains. While these powerful methodologies have seen profound success, there exists specific limitations to real-world DoD use cases. These include brittleness in dynamic environments, requirements for “expensive” training data, inability to update classification and prediction regimes in near-real time, and difficulty trusting deep net solutions during critical failure modes. The operationalization of machine learning approaches within the DoD will require some assurances that these complex computational methods are obeying the commander’s intent and provide an explainable framework that seamlessly integrates with human decision making. For this reason, we have developed an explainable AI approach (XAI) that utilizes the underpinnings of topological data analysis, using the mapper algorithm, to hierarchically organize knowledge representations of multiple domain data types. In this talk, we describe a topological hierarchical decomposition (THD) method that architects information based on robust feature similarity across covering parameters. We have begun to utilize this THD functional unit to generate higher order architectures, where THDs can be utilized for feature exploration, feature synthesis, classification and prediction, and multi-modal abstraction. By using the overall output activation across a number of THDs as inputs for successive THD layers, deep topological networks can be constructed. We will demonstrate the construction of one of these unsupervised deep topological networks using the MNIST dataset, discuss recent work in utilizing gradient descent and feedback alignment approaches for supervision, and highlight multiple applications of the approach in domains such as satellite imagery classification tasks, wide-area motion object tracking, radio frequency modulation prediction, and mining DNN architectures for explainability and classification performance enhancement.