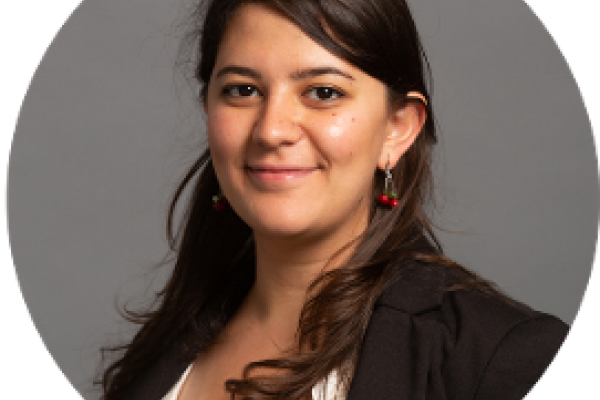
Title: Breaking isometric ties and incorporating feature priors in Gromov-Wasserstein distance for single-cell multi-omic integration
Speaker: Pınar Demetçi (Broad Institute of MIT and Harvard)
Speaker's URL: https://pinardemetci.github.io/
Abstract: Gromov-Wasserstein (GW) distance has found many applications in various fields due to its ability to compare measures across metric spaces. As GW considers intra-domain pairwise distances, it is naturally invariant to isometric transformations. While its invariance is a blessing in some applications, in others, it can be too flexible and undesirable. Moreover, by disregarding raw features, it eliminates the possibility of incorporating any prior information that may exist on feature associations. We extend GW to Augmented Gromov Wasserstein (AGW) by proposing an interpolation with CO-Optimal Transport. We demonstrate that AGW allows for some control over the level of rigidity to isometric transformations and leads to improved object comparison in downstream applications. In particular, we apply AGW to the single-cell multi-omic data alignment task in computational biology. In this task, we match data points from biological experiments that measure different aspects of the genome to overcome experimental difficulties of co-measurements and enable multi-modal analysis. AGW outperforms the existing optimal transport-based approaches and yields increased performance with sample-level (i.e. cells) or feature-level (i.e. genes) supervision.
URL associated with Seminar: https://tgda.osu.edu/