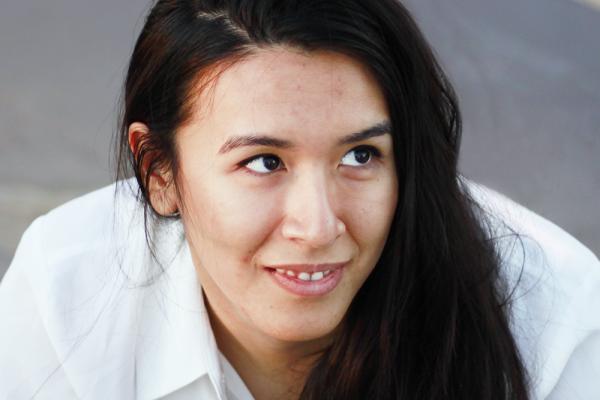
Title: High fidelity numerical codes: structure-preserving schemes with data-driven models
Speaker: Maria Han Veiga (University of Michigan)
Speaker's URL: https://hanveiga.com/
Abstract: Many engineering and scientific problems can be described by equations of fluid dynamics, namely, systems of time dependent nonlinear hyperbolic PDEs. The mathematical description of these processes as well as the numerical discretisation of the resulting PDEs will depend on the level of detail required to study them.
In this talk I will focus on two directions towards higher fidelity numerical simulations: first, I present a novel structure-preserving arbitrarily high-order method that solves the nonlinear ideal magneto-hydrodynamics equations. Secondly, I will focus on our work using Machine Learning (ML) methods with the aim to improve or speed up numerical simulations, through the development of parameter-free routines as part of a numerical solver or surrogate models, with the goal of creating hybrid simulation pipelines that can improve over time.
URL associated with Seminar: https://people.math.osu.edu/xing.205/seminar.html