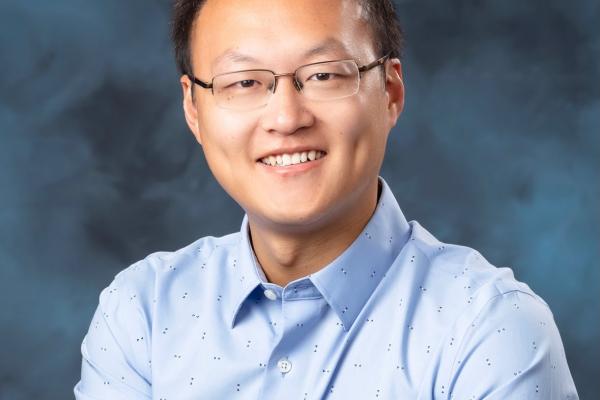
Title: A Nonlocal Gradient for High-Dimensional Black-Box Optimization in Scientific Applications
Speaker: Guannan Zhang (Oak Ridge National Laboratory)
Speaker's URL: https://sites.google.com/view/guannan-zhang/
Abstract: In this talk, we consider the problem of minimizing multi-modal loss functions with a large number of local optima. Since the local gradient points to the direction of the steepest slope in an infinitesimal neighborhood, an optimizer guided by the local gradient is often trapped in a local minimum. To address this issue, we develop a novel nonlocal gradient to skip small local minima by capturing major structures of the loss's landscape in black-box optimization. The nonlocal gradient is defined by a directional Gaussian smoothing (DGS) approach. The key idea is to conducts 1D long-range exploration with a large smoothing radius along orthogonal directions, each of which defines a nonlocal directional derivative as a 1D integral. Such long-range exploration enables the nonlocal gradient to skip small local minima. We use the Gauss-Hermite quadrature rule to approximate the d 1D integrals to obtain an accurate estimator. We also provide theoretical analysis on the convergence of the method on nonconvex landscape. In this work, we investigate the scenario where the objective function is composed of a convex function, perturbed by a highly oscillating, deterministic noise. We provide a convergence theory under which the iterates converge to a tightened neighborhood of the solution, whose size is characterized by the noise frequency. Furthermore, if the noise level decays to zero when approaching global minimum, we prove that the DGS optimization converges to the exact global minimum with linear rates, similarly to standard gradient-based method in optimizing convex functions. We complement our theoretical analysis with numerical experiments to illustrate the performance of this approach.