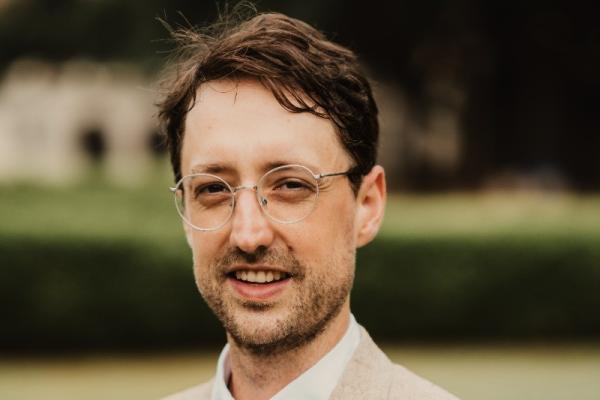
Thomas O'Leary-Roseberry
University of Texas at Austin
Title
Derivative-Informed Scientific Machine Learning
Abstract
Decision-making for complex physical systems is a core aspect of modern engineering. By employing computational models, engineers can explore many questions related to system design and control. However, because observations and computational models both contain inherent errors and uncertainties, robust statistical models must be developed. Such models enable (i) the statistical calibration of computational models to noisy observational data, and (ii) the formulation of risk-averse optimization strategies for system management. A major computational challenge arises because these statistical models often require repeated evaluations of expensive computational models for various input parameters.
In this talk, we present ways to construct advanced machine learning approximations of high-fidelity computational models by leveraging the derivative information of model outputs with respect to their inputs. Our approaches (1) use this derivative information to identify low-dimensional subspaces that yield efficient dimension-reduced surrogate models, and (2) incorporate derivatives into training to produce accurate surrogates for derivative-based inference and optimization. These derivative-informed neural operators yield efficient, scalable, and state-of-the-art algorithms for Bayesian inverse problems and for optimal design and control of complex systems. Our methods are supported by rigorous analyses, including a priori error bounds for optimization, and demonstrate exceptional performance in numerical experiments. We show their effectiveness in various mechanics problems, such as inverse problems in solid deformation for part qualification and the inversion of material properties beneath Japan using data from the 2011 Tohoku earthquake, as well as in fluid flow control. In all these cases, these models deliver significantly higher accuracy per unit compute than standard surrogates, resulting in orders-of-magnitude speedups in inverse and optimization tasks when compared to conventional numerical PDE models.
Time permitting, we will also explore sample-efficient algorithms for stochastic optimization problems, which arise in the aforementioned tasks. These problems are common in machine learning and computational mathematics and form a large part of modern computing workloads. We present Hessian-averaging methods that employ adaptive gradient sampling and incur a fixed per-iteration Hessian costs. We establish very fast local superlinear convergence rates while accommodating inexact Hessian and gradient estimates to ensure computational efficiency. Numerical results on challenging deep learning tasks demonstrate both the practical and theoretical benefits of these methods.
Short Bio:
Dr. Thomas O'Leary-Roseberry is a Research Associate in the OPTIMUS Center of the Oden Institute at The University of Texas at Austin. He received his undergraduate degrees in Mathematics and Engineering Mechanics from the University of Wisconsin--Madison in 2015, and his PhD in Computational Science, Engineering, and Mathematics from The University of Texas at Austin in 2020. He works on numerical methods for the inference, prediction, and optimal design and control of complex physical systems which are often mathematically modeled as parametrized partial differential equations (PDEs). His work blends numerical analysis, machine learning, and mechanistic modeling to create state-of-the-art methods to support decision making regarding complex systems under high-dimensional uncertainty.