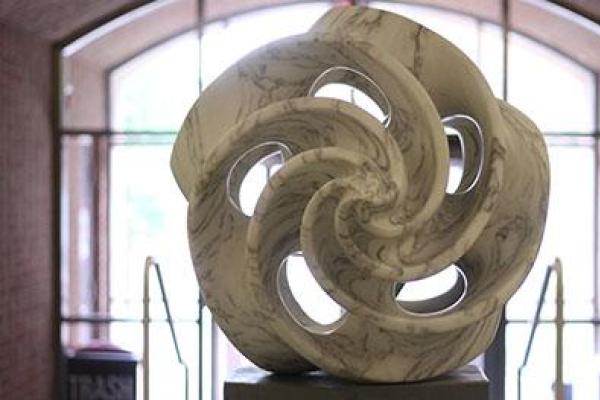
Speaker: Chunmei Wang
Title: Decoding Data: The Finite Expression Method as a Symbolic Approach for Scientific Machine Learning
Abstract: Mathematical modeling from experimental data is an important and challenging problem in diverse applications in science and engineering. In this talk, we introduce the "Finite Expression Method" (FEX), a deep symbolic learning technique, to discover interpretable and explicit mathematical models with finitely many operators from observed data. FEX is a flexible framework with a wider range of applications, higher accuracy, and better noise robustness than many existing symbolic learning approaches. In the context of mathematical modeling on complex networks, a fast training algorithm based on stochastic sampling is proposed to accelerate the training of FEX, improving the computation cost to a much smaller scale. Various numerical and theoretical results will be provided to justify the performance of FEX. A future agenda of FEX for general scientific machine learning problems will also be discussed.