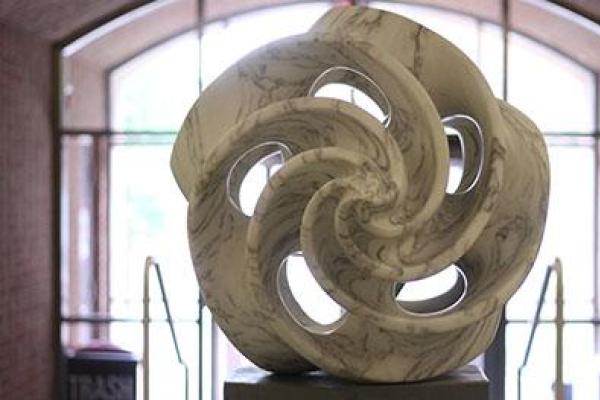
Speaker: Chudamani Poudyal
Title: Robust and Efficient Predictive Models for Actuarial Claim Severity Data
Abstract: Predictive models are designed to predict the cost of uncertain future claims. Insurance and financial claims are usually non-negative number, for example, automobile, liability, or operational risk insurance, etc. There are three challenges when constructing a probabilistic model. They are (i) sample data are often affected by the presence of deductibles, policy limits and coinsurance, (ii) past data may contain outliers (unusual observations that can distort the results), and (iii) a need to evaluate the accuracy of the forecast. The contemporary literature in this area treats the three items as separate problems, leaving the practitioner with an incomplete framework for model building and assessment. In this presentation, we provide a comprehensive framework for addressing all three of these challenges simultaneously mainly via three estimation procedures: (a) maximum likelihood estimator (MLE), adaptive (b) method of fixed tail probabilistic moments (MTM & MWM), and (c) method of random tail probabilistic moments (MTuM & variants). Asymptotic distributional properties are established (along with extensive simulation studies) which are essential parts for statistical inferences. Numerical examples will be provided for 1500 US Indemnity Losses which illustrate the practical performance of the established results. Future directions in designing machine learning algorithms will be discussed.