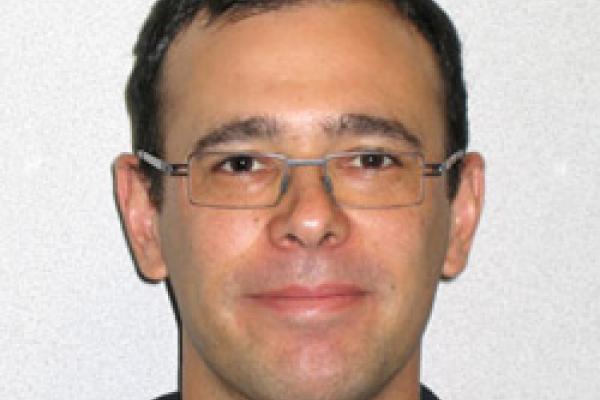
Title: Metric geometry and data analysis
Speaker: Facundo Memoli, The Ohio State University
Seminar Type: WELCOME
Abstract: In many problems arising in the computational sciences datasets come equipped with some underlying notion of similarity. Metric geometry provides a natural point of view which is applicable in the realm of non-smooth data.
One idea is to represent datasets as metric measure spaces and then, at the same time, regard the collection D of all datasets as a metric space in itself. The Gromov-Wasserstein distance --a variant of the Gromov-Hausdorff distance based on ideas from mass transport-- provides an intrinsic metric on D which exhibits non-negative curvature in the Alexandrov sense.
I will describe the construction of the GW metric, its properties, and discuss how the representation of datasets as metric measure spaces gives rise to a number of stable invariants which are counterparts to concepts emerging in algebraic topology and differential geometry, including analogues to homology and notions of curvature that are valid beyond the smooth case.